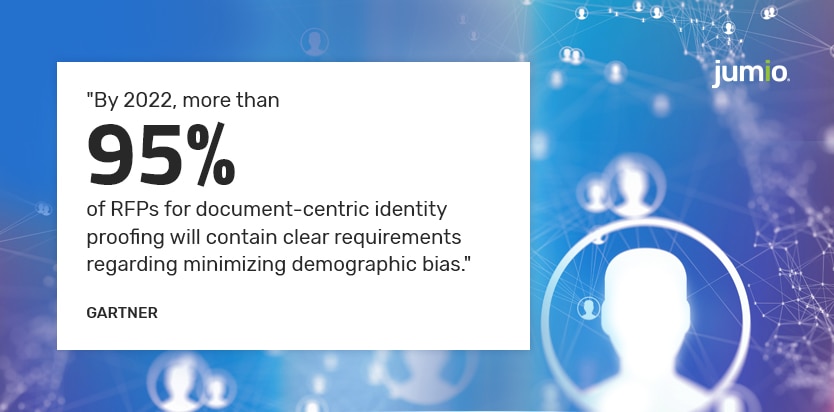
AI and racial bias seem to be increasingly intertwined these days.
When bias becomes embedded in machine learning models, it can have an adverse impact on our daily lives. The bias is exhibited in the form of exclusion, such as certain groups being denied loans or not being able to use the technology, or in the technology not working the same for everyone. As AI continues to become more a part of our lives, the risks from bias only grow larger.
In the context of facial recognition, racial bias is only one form of bias. Other demographic traits, such as age, gender, socioeconomic factors, and even the quality of the camera/device can impact software’s ability to compare one face to a database of faces. In these types of surveillance, the quality and robustness of the underlying database is what can fuel bias in the AI models. Modern facial recognition software uses biometrics to map facial features from a photograph or video. It then compares the information with a database of known faces to find a match (this is known as a 1:n match).
The American Civil Liberties Union (ACLU) studied Amazon’s AI-based Rekognition facial recognition software back in 2018 and found that Rekognition falsely matched 28 U.S. Congress members with a database of criminal mugshots. According to the ACLU, “Nearly 40 percent of Rekognition’s false matches in our test were of people of color, even though they make up only 20 percent of Congress.”
But, demographic bias isn’t just an issue for facial recognition — it’s also an issue for facial authentication which relies on the unique biological characteristics of an individual to verify that she is who she claims to be.
Facial recognition and facial authentication, however, are two very different kinds of animals.
Most leading identity verification solutions leverage AI and machine learning to assess the digital identity of remote users — and, unfortunately, these algorithms are also susceptible to demographic bias which include race, age, gender and other characteristics. But, this type of bias has nothing to do with the underlying database because this type of authentication doesn’t perform 1:n-type searches against an established database of images.
It’s a whole different kind of AI that is brought to bear to solve a very different business problem — if the person is who they claim to be when creating new accounts online.
AI algorithms are used to compare the selfie of a customer with the photo in their identity document. According to Gartner, “there has always been awareness of possible bias in this facial recognition process. However, we have observed clients showing far greater interest in this topic during the past six months. This is probably due to the increased political narrative and discussion on different aspects of inequality driven by the Black Lives Matter movement.”
Strategic Planning Assumption
By 2022, more than 95% of RFPs for document-centric identity proofing will contain clear requirements regarding minimizing demographic bias, an increase from fewer than 15% today.
2020 Gartner Market Guide for Identity Proofing and Affirmation
Bias can creep into algorithms in several ways. AI systems learn to make decisions based on training data, which can include biased human decisions or reflect historical or social inequities, even if sensitive variables such as gender, race or sexual orientation are removed.
Here are five critical questions you can ask would-be solution providers to determine how well they are addressing demographic bias:
1. How big and representative is your training database?
AI training data is the information used to train a machine learning model. Machine learning models use the training dataset to learn how to recognize patterns and apply technologies such as neural networks, so that the models can make accurate predictions when later presented with new data in real world applications. When it comes to AI, size matters. The larger and more representative the training data set, the better its ability to withstand the introduction of demographic bias.
For example, popular voice assistants such as Siri or Alexa that are trained on huge databases of recorded speech that are unfortunately dominated by speech from white, upper-middle class Americans. This makes it challenging for the technology to understand commands from people outside that category. These voice assistants are also susceptible to different accents where Western accents have a higher recognition that others.
This lack of representation is what leads to biased datasets and ultimately algorithms that are much more likely to perpetuate systemic biases. Similarly, think about a face detection model that is trained on a large dataset of faces from a single ethnicity. It will most likely fail to detect faces from another ethnicity. If you’re building algorithms for Romanian IDs, it helps to have tens of thousands of Romanian IDs versus hundreds of ID documents to build algorithms that can better detect fraud and find anomalies.
2. Where did the data come from to create the training data sets?
When companies don’t have enough of their own data to build robust models, they often turn to third-party data sources to backfill this gap, and these purchased datasets can introduce unintentional bias. For example, a dataset of images of ID documents captured under perfect lighting conditions with high-resolution cameras is not representative of ID images that are captured in the real world. Not surprisingly, AI models built on unrealistic models will struggle with IDs that contain blur or glare or were captured in dim lighting. Algorithms that were built with real-world production data, on the other hand, will contain documents with real-world imperfections. As a result, these AI models are more robust and less susceptible to demographic bias.
3. How were the data sets labeled?
In most AI projects, classifying and labeling data sets takes a fair amount of time, especially with enough accuracy and granularity to meet the expectations of the market. In the context of identity verification, labeling is how the ID documents are tagged. If the photo of the ID has been manipulated, then the document will be tagged as fraudulent with photo manipulation. If the picture of the ID has excessive glare, blur or was captured in poor lighting, then the labels should reflect those characteristics. If the wrong labels are used when tagging individual identity verification transactions, the AI models will bake that information into the algorithms which will make the models less accurate and more subject to bias.
Some solution providers outsource or crowdsource the tagging exercise using solutions like Amazon’s Mechanical Turk. Other solutions insource the image tagging to experienced agents who are instructed how to tag verification transactions to optimize the learning curve of the AI models. Naturally, the insourcing models generally result in more accurate models being developed.
4. What type of quality controls are in place to govern the tagging process?
Unfortunately, a lot of this bias is unconscious because many solution providers do not necessarily know when they’re making the algorithm that it’s going to make incorrect outcomes. That’s why there needs to be some quality control injected into the process. In the identity verification space, there’s no substitute for having a trained crew of tagging specialists who know how to accurately tag individual ID transactions and auditing processes in place to check their work.
5. How diverse is the team developing the algorithms?
Reducing bias is also about the people who are developing the AI algorithms and tagging the datasets. It’s not unfair to ask about the composition of the AI team. Ideally, the AI engineers and data scientists come from a variety of nationalities, genders, ethnicities, professional experiences and academic backgrounds. This diversity helps ensure that different perspectives are brought to bear on the models being created which can help reduce some demographic bias.
There is a growing concern that demographic bias in a vendor’s AI models could reflect negatively on a company’s brand and possibly raise possible legal issues, especially when economic decisions are dependent upon the accuracy and reliability of those algorithms. Believe it or not, these algorithms can result in some types of customers being unfairly rejected or discounted, which translates to lost business and downstream opportunities. That’s why it’s increasingly important to understand how vendors measure demographic bias and what measures they are taking to address it.
To see how Jumio addresses demographic bias within our AI processes, check out our new reference guide.